Not everyone you disagree with on social media is a bot, but various forms of social media manipulation are indeed used to spread false narratives, influence democratic processes and affect stock prices.
In 2019, the global cost of bad actors on the internet was conservatively estimated at $78 billion. In the meantime, misinformation strategies have kept evolving: Detecting them has been so far a reactive affair, with malicious actors always being one step ahead.
Alexander Nwala, a William & Mary assistant professor of data science, aims to address these forms of abuse proactively. With colleagues at the Indiana University Observatory on Social Media, he has recently published an Open Access paper in EPJ Data Science to introduce BLOC, a universal language framework for modeling social media behaviors.
“The main idea behind this framework is not to target a specific behavior, but instead provide a language that can describe behaviors,” said Nwala.
Automated bots mimicking human actions have become more sophisticated over time. Inauthentic coordinated behavior represents another common deception, manifested through actions that may not look suspicious at the individual account level, but are actually part of a strategy involving multiple accounts.
However, not all automated or coordinated behavior is necessarily malicious. BLOC does not classify “good” or “bad” activities but gives researchers a language to describe social media behaviors — based on which potentially malicious actions can be more easily identified.
A user-friendly tool to investigate suspicious account behavior is in the works at William & Mary. Ian MacDonald ’25, technical director of the W&M undergraduate-led DisinfoLab, is building a BLOC-based website that would be accessed by researchers, journalists and the general public.
Checking for automation and coordination
The process, Nwala explained, starts with sampling posts from a given social media account within a specific timeframe and encoding information using specific alphabets.
BLOC, which stands for “Behavioral Languages for Online Characterization,” relies on action and content alphabets to represent user behavior in a way that can be easily adapted to different social media platforms.
For instance, a string like “TpπR” indicates a sequence of four user actions: specifically, a published post, a reply to a non-friend and then to themselves and a repost of a friend’s message.
Using the content alphabet, the same set of actions can be characterized as “(t)(EEH)(UM)(m)” if the user’s posts respectively contain text, two images and a hashtag, a link and a mention to a friend and a mention of a non-friend.
The BLOC strings obtained are then tokenized into words which could represent different behaviors. “Once we have these words, we build what we call vectors, mathematical representations of these words,” said Nwala. “So we’ll have various BLOC words and then the number of times a user expressed the word or behavior.”
Once vectors are obtained, data is run through a machine learning algorithm trained to identify patterns distinguishing between different classes of users (e.g., machines and humans).
Human and bot-like behaviors are at the opposite ends of a spectrum: In between, there are “cyborg-like” accounts oscillating between these two.
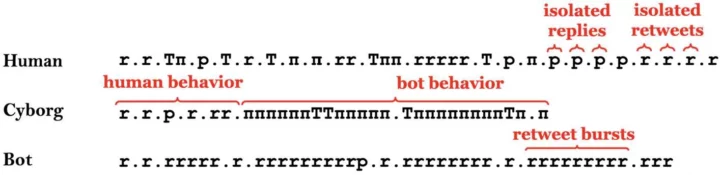
“We create models which capture machine and human behavior, and then we find out whether unknown accounts are closer to humans, or to machines,” said Nwala.
Using the BLOC framework does not merely facilitate bot detection, equaling or outperforming current detection methods; it also allows the identification of similarities between human-led accounts. Nwala pointed out that BLOC had also been applied to detect coordinated inauthentic accounts engaging in information operations from countries that attempted to influence elections in the U.S. and the West.
“Similarity is a very useful metric,” he said. “If two accounts are doing almost the same thing, you can investigate their behaviors using BLOC to see if perhaps they’re controlled by the same person and then investigate their behavior further.”
BLOC is so far unique in addressing different forms of manipulation and is well-poised to outlive platform changes that can make popular detection tools obsolete.
“Also, if a new form of behavior arises that we want to study, we don’t need to start from scratch,” said Nwala. “We can just use BLOC to study that behavior and possibly detect it.”
Beyond online bad actors
As Nwala points out to students in his class on Web Science — the science of decentralized information structures — studying web tools and technologies needs to take into account social, cultural and psychological dimensions.
“As we interact with technologies, all of these forces come together,” he said.
Nwala suggested potential future applications of BLOC in areas such as mental health, as the framework supports the study of behavioral shifts in social media actions.
Research work on social media, however, has been recently limited by the restrictions imposed by social media platforms on application programming interfaces.
“Research like this was only possible because of the availability of APIs to collect large amounts of data,” said Nwala. “Manipulators will be able to afford whatever it takes to continue their behaviors, but researchers on the other side won’t.”
According to Nwala, such limitations do not only affect researchers, but also the society at large as these studies help raise awareness of social media manipulation and contribute to effective policymaking.
“Just as there’s been this steady shout about how the slow decline of local news media affects the democratic process, I think this rises up to that level,” he said. “The ability of good faith researchers to collect and analyze social media data at a large scale is a public good that needs not to be restricted.”
Editor’s note: Data and democracy are two of four cornerstone initiatives in W&M’s Vision 2026 strategic plan. Visit the Vision 2026 website to learn more.
Antonella Di Marzio, Senior Research Writer